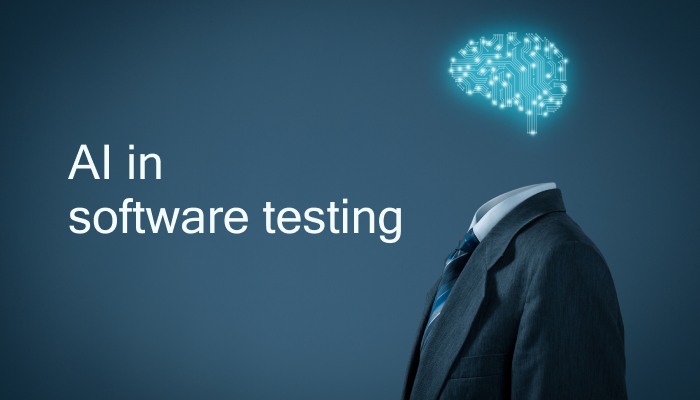
Whether you aim to launch your first piece of software onto the global market or are already engaged in multiple development or testing contracts, artificial intelligence (AI) algorithms can make your job a lot easier. According to DZone, up to 70% of software testing can be performed by AI at any given moment, with 30% being reserved for social engineering and user-centric scenario tests.
The same data implies that 64% of companies will integrate AI into their quality assurance (QA) in order to improve customer servicing processes in the near future. With the rising demand for high-quality software solutions in a plethora of industries and niches, AI can not only fast-track your development cycle but also ensure that the majority of bugs and glitches are ironed out pre-launch. With that said, let’s dive into some of the minute reasons of why you should integrate AI into software testing going forward.
AI in Software Testing 101
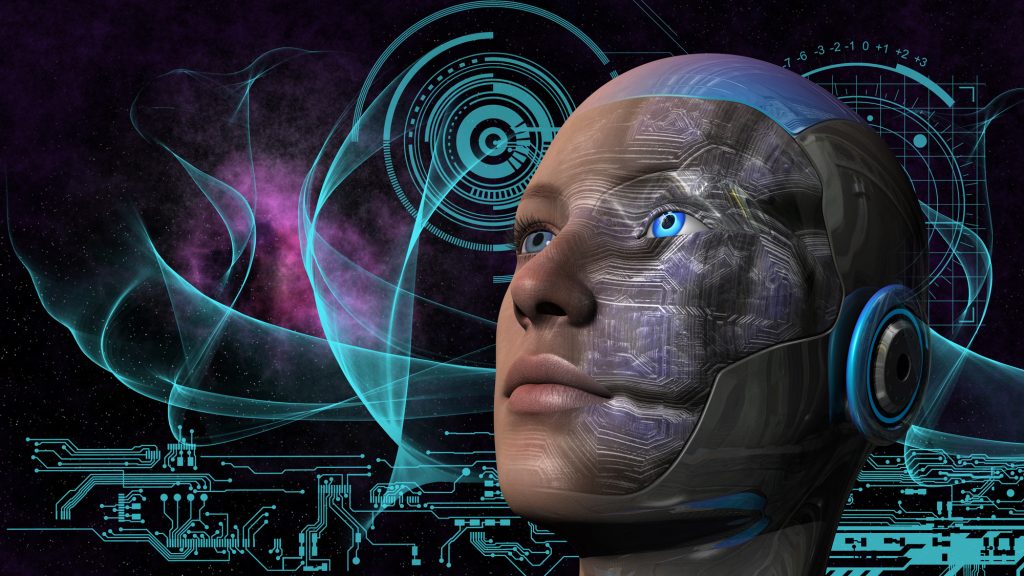
Before we go any further, let’s discuss what AI represents in the lens of software testing. Software testing is a process in which developers and testers use the front-end elements of a newly-designed piece of software in order to test its functionality.
Cynthia Malik, QA Specialist at Studicus put it this way: “Every time you add a new line of code to your application, you want to make sure that it doesn’t cancel out or prohibit an existing function from executing properly. Even though manual QA is always welcome, the addition of AI can make the process faster and more accurate, especially in the long weeks or months before the actual launch.” Artificial intelligence algorithms present in the software testing industry are designed to perform the same steps and calls as a manual QA expert.
The difference between the two should be obvious, given that one is driven by logic and the other by expertise and experience. However, neither should become the sole channel for software testing as one can always detect a small discrepancy the other wouldn’t be able to. Finding the right balance between manual and AI-driven software testing is what will truly enhance and enrich the final product.
Elimination of Manual Testing Limits
Manual software testing is typically limited by the performance and productivity of individual QA testers. As such, introducing AI testing into your QA environment will ensure that these limits are eliminated going forward. Things such as work hours, prolonged testing cycles and wait times due to manual data collection and submission become an afterthought with AI in the mix.
The speed at which artificial intelligence can cover software code and extrapolate testing data cannot be reached by hand, even if a team of QA testers is involved in the singular testing process. This is especially important in software development environments with multiple ongoing projects and short deadlines, making manual testing nigh impossible. If you experience issues with breaches of deadlines and bottlenecks due to extended manual testing times, make sure to give AI algorithms a shot.
Short Turnaround Times
Due to the nature of software development and an increased focus on agile workflow, applications are never really “done” until the final deadline. However, short turnaround times can also bring forth a plethora of issues and bugs in the code published as the final product. James Tanner, Data Analyst at WoWGrade spoke on the matter recently: “Developing an AI-based QA testing environment is highly beneficial when it comes to projects with short development cycles which leave very little room for manual software testing apart from baseline functionality tests.”
Failing to add AI algorithms to short software development cycles can not only harm the final product but also cost your team its reputation and trust in terms of future contracts. As we’ve previously stated, finding the sweet-spot between manual testing and AI implementation will ensure that errors are stamped out on time and that the final product meets both your clients’ and users’ standards.
Improved Data Accuracy
While there is no denying that experienced QA testers and data analysts can stamp out bugs in software code with ease and precision, human error is bound to happen sooner or later. When it comes to AI software testing however, data accuracy is an afterthought and a given. AI algorithms never make mistakes when it comes to software testing due to their very nature as a logical mechanism.
Once you set up the testing environment and run your AI to go through all the variations on a piece of software, you can rest assured that its extrapolated data will be as precise as it is possible in the industry. This data can be further complemented by allowing your QA testers to scan through the data and make sure that everything is in order, which in turn takes less time than fully manual testing.
Constant Objectivity
Just like in any other industry or life situation, we are all guilty of “if this then that” mentality. The same rule applies for QA testers, developers and data analysts assigned to software testing. Human error can extend further than data accuracy, leading to misunderstanding and confusion with how a piece of software is supposed to perform.
Thomas Silva, Head of Content Development at Trust My Paper had this to say on the subject: “Creativity and objectivity rarely go hand in hand – this is especially true in content and software development. While the former can be fully managed by real-world specialists, objective data analysis should be delegated to artificial intelligence in order to draw the best from both worlds.”
Better Testing Coverage
Software testing can cover either a specific set of parameters or the entirety of an application’s code. In the latter case, manual testing is ill-advised for numerous reasons. As stated in the DZone article we’ve discussed previously, user-centric software testing should focus on scenarios typically associated with social engineering.
Software application uses which would be taken by human users should be handled by real-world QA testers while everything else from baseline to advanced functionality can be delegated to automated algorithms. This can create an environment where testing coverage is much better than in case of fully manual workflow, thus eliminating any chances of errors or bottlenecks slipping under the radar.
High Return on Investment
Lastly, AI in software testing can yield a very high return on investment (ROI) compared to QA testers. Once AI algorithms are defined and ready to run, their repeated operations won’t cost any additional resources. Compared to real-world specialists which require, time and monetary compensation (in addition to a healthy work environment), AI can prove highly efficient and economical for your development team.
Bruce Thames, Head of QA at IsAccurate spoke on the matter briefly: “You should never fully eliminate human testers in your QA processes despite the advancements in AI technology in this sector. However, by shifting the balance of resources to the AI side of the equation, you can save both time and resources, delivering a high-quality product faster and with lesser costs for your business.”
The Future is Artificial (Conclusion)
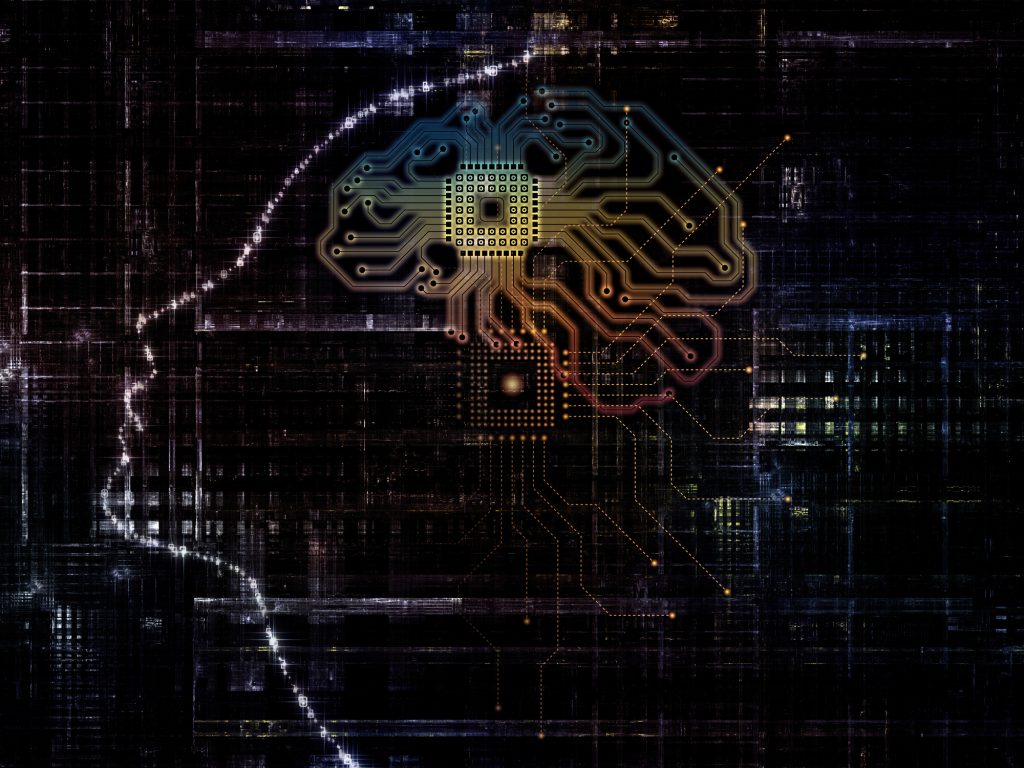
As we move forward and closer to 2020, AI algorithms in numerous fields such as software testing and chatbot are bound to take precedence over human agents. Even though AI still cannot compete with real-world specialists, their continued presence in the software development field is undoubtedly creating waves in the industry at large.
Find a creative, beneficial and resource-friendly way to integrate AI into your software testing environment without sacrificing the human element. With time, you can develop a great combination of the two which will maximize your team’s performance while also eliminating unnecessary downtime and bottlenecks in the software testing process.
Dorian Martin is a frequent blogger and an article contributor to a number of websites related to digital marketing, AI/ML, blockchain, data science and all things digital. He is a senior writer at Supreme Dissertations, runs a personal blog NotBusinessAsUsusal and provides training to other content writers.